.webp)
Published onÂ
June 3, 2025
Fraud Detection Rules: Types, Benefits, and Selection Tips
In this story
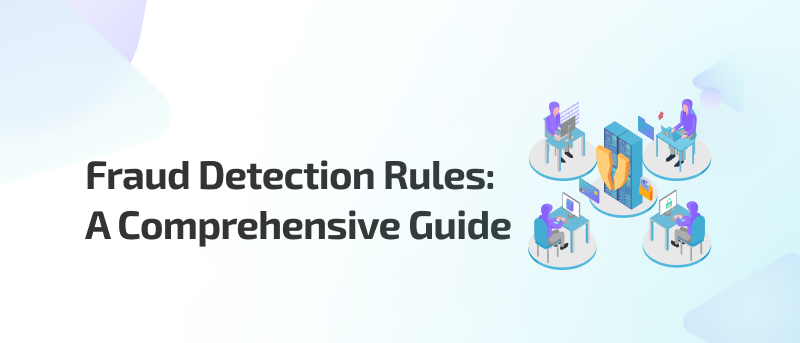
Accelerate AML Compliance: Meet Regulatory Demands with 80% Less Setup Time
According to the Association of Financial Professionals, fraudulent activities impacted 82% of businesses in 2018 alone. Research predicts a staggering total fraud loss of $362 billion between 2023 and 2028. This means that fraudsters are always coming up with new ways to manipulate the system. But having a strong fraud prevention strategy can actually turn the situation upside down.
What Are Fraud Detection Rules?
Fraud detection rules are essential if/then statements integral to risk management technology, aiding in decision-making. These rules work by setting conditions, like checking chargeback history or connecting device emails with IP addresses, and even verifying age or ensuring content follows the rules.
In other words, we can say that a fraud detection rule is a condition that relies on data to determine the legitimacy of an activity, enabling the review, approval, or declination of user actions. For instance, a basic rule might block website access if an IP address is flagged as fraudulent.
Rule-based fraud detection spans from simple, static rules to intricate velocity checks, assessing actions within specific time frames.
Equip yourself with the knowledge and insights needed to combat fraud effectively in our practical Fraud Prevention E-Book
How Do Fraud Detection Rules Work?
Fraud detection rules operate in two main ways: with machine learning and without machine learning, each serving distinct purposes. In scenarios involving machine learning, the process involves data collection during user interactions, activation of machine learning for risk assessment, consultation of predefined rules, and automated decision-making aligned with those rules.
On the other hand, situations without machine learning, though usually temporary, rely on predefined rules for decision-making based on factors like geographical restrictions, emerging threats, or evolving risk thresholds.
Comply quickly with local/global regulations with 80% less setup time
Types of Fraud Detection Rules
Fraud management rules serve as crucial components in decision-making processes. They enable businesses to proactively detect and reduce potential fraud risks. Within a rule-based fraud detection system, not all rules are identical. Below, we discuss five distinct types of fraud detection rules:
1. Static Rules for Fraud Detection
- Basic if/then logic, often blocking user actions.
- Historical use related to IP addresses, but it can be creative based on available data.
- Notably lacks flexibility, leading to potential false positives.
2. Scoring Rules for Fraud Detection
- Inform risk strategy without necessarily blocking actions.
- Fraud scores are adaptable to business needs and can automate prevention based on thresholds.
3. Velocity Rules for Fraud Detection
- Assess user behavior through set actions over a specific time period, for instance, monitoring login attempts within a defined timeframe to prevent account takeover fraud.
4. Machine Learning Rules for Fraud Detection
- Built on artificial intelligence, learns from previous fraud cases.
- Analyzes extensive data to identify emerging suspicious patterns.
- Provides personalized suggestions based on learned insights.
8 Benefits of a Rules-Based Fraud Detection Solution
To begin with, a rules-based fraud detection solution offers a range of benefits. In this section, we will explain the top eight benefits of employing rules-based fraud detection solutions to conduct comprehensive fraud investigations:Â
1. Efficiency
- Quick and easy setup of rules-based fraud detection solutions.
- Immediate scanning of all transactions in real-time for potential fraud.
2. Simplicity
- Rules-based systems are transparent and easy to interpret.
- Facilitates quick identification and resolution of issues, ensuring minimal business disruption.
3. Instant Response to Threats
- Rapid insertion of rules to counteract emerging fraud trends.
- Allows fraud analysts to swiftly stop ongoing attacks, such as location-based blacklisting.
4. Proactive Blocking of New Fraud Trends
- Rules enable proactive prevention of fraud before machine learning models adapt to emerging trends.
- Specific rules tailored to known characteristics of fraud behavior help target the right patterns.
5. Allowing Good Customers
- Rules can be used not just to prevent fraud but also to allow specific customer behaviors.
- Facilitates collaboration with other business departments, such as marketing, by allowing specific promotions.
6. Safeguards against Misconfigurations
- Safeguards prevent misconfigured rules that could harm the business.
- Impact tests ensure a reasonable estimate of the rule's effects on user data, with safeguards in place for impactful changes.
7. Scalability and Automation
- Enables scaling of fraud prevention strategies through automated checks based on rules.
- Allows dynamic friction, permitting manual review of cases within a grey area to avoid blocking legitimate users outright.
8. Reduction of Fraud Rates Over Time
- A data-driven approach facilitates monitoring results, setting KPIs, and adjusting rules for continuous improvement.
- Integration of machine learning provides relevant suggestions based on the unique business use case.
How to Choose the Best Rule-Based Fraud Detection Solution?
Indeed, choosing a solution to fraud management rules involves critical considerations, and evaluating the quality of a vendor's rules and machine learning capabilities is crucial. However, when considering the below mentioned criteria, you will make sure you’re choosing the best rule-based fraud prevention solution for your business needs.
1. Effectiveness
- Assess whether the solution yields the desired results.
- Evaluate the approach, whether it relies on shared blacklists or real-time data, to ensure it aligns with your fraud prevention strategy.
2. Flexibility
- Seek a solution that offers flexibility in rule customization.
- Explore options that allow stacking rules, utilizing fraud scoring, custom fields, and even integrating machine learning suggestions for long-term benefits.
- Consider the adaptability of the solution to your specific vertical, recognizing that different industries may have unique requirements.
3. Scalability
- Examine the scalability of the system by determining the number of rules it can support.
- Evaluate the system's capability to handle a high volume of API calls per day, which is crucial for analyzing transactions at scale.
- If hosted online, consider the uptime rate to ensure uninterrupted fraud detection capabilities.
4. Cost Considerations
- Analyze the cost structure, whether it involves payment per API call or a lump sum for a multiyear contract.
- Consider factors such as micro fees versus chargeback guarantees to make an informed financial decision. In fact, chargeback fraud is one of the most common types of fraud.
5. Ease of Integration
- Assess how easy it is to integrate the solution with your existing platform.
- Consider the time and effort required for a seamless integration process, ensuring minimal disruption to your operations.
6. Quality of Rules and Machine Learning
- Look for solutions that offer customizable rules, recognizing that no two businesses are identical.
- Ensure that the platform allows for rule changes promptly and without complexity.
- Consider the depth of data analysis beyond risk scores, as accurate decisions should be based on comprehensive data evaluation.
7. Managing Fraud and Risk
- Verify that the solution incorporates rules designed to manage both fraud and nonfraud risks.
- Recognize the distinction between fraud and risk rules, understanding that compliance issues can lead to financial loss in the long run.
8. Immediate Impact and Data Backed Decisions
- Confirm that rule changes take effect immediately, acknowledging the urgency of responding to emerging fraud threats.
- Ensure that decisions are backed by the right data, focusing on data quality and diversity rather than relying solely on interaction count metrics.
9. Machine Learning Advancements
- Scrutinize machine learning capabilities, seeking platforms that offer tailor-made models for your business without requiring extensive manual efforts.
- Evaluate claims about customized solutions, understanding whether the technology is built to self-optimize over time or necessitates manual processes for training.
How Can FOCAL Rule-Based Fraud Prevention Solution Help?
In fact, FOCAL fraud prevention solution enables businesses to experience a solution that allows them to score, comprehend, and monitor fraud and risks with unparalleled flexibility. It is designed to adapt to your company's specific requirements.
- Flexible Deployment Options: Implement the solution swiftly and seamlessly without causing disruptions to your operations.
- Industry-Specific Risk Rules: Initiate risk rules tailored to your industry by drawing insights from similar fraud cases.
- Customization Excellence: Fully customize risk rules to align precisely with the unique needs of your business.
- Powerful AI engines and capabilities: Receive valuable suggestions from a strong machine-learning and AI engine for enhanced decision-making and identifying as well as addressing new fraud patterns effectively.
- Effortless Rule Management: Easily add, edit, activate, or deactivate rules with a user-friendly interface.
- Seamless And Simple API Integration: Seamlessly integrate the solution into your existing systems with a high degree of flexibility.
- Payment Flexibility: Enjoy the same level of flexibility in payment options, ensuring a tailored approach to your business needs.
Conclusion
In conclusion, a rule-based fraud detection solution should meet current requirements and provide the flexibility and scalability to adapt to evolving fraud prevention needs. It should be a dynamic, cost-effective solution that aligns with your business goals and risk management strategies.
FAQs
Q1. What Does A Rule Engine For Fraud Detection Entail? Â
A rule engine for fraud detection involves a system that utilizes predefined if/then statements to make decisions in identifying and preventing fraudulent activities.
Q2. Why Opt For A Rules-Based Fraud Prevention Solution? Â
Rules-based solutions offer flexibility, rapid deployment, and customization, allowing tailored strategies to combat fraud in various industries.
Q3. What Is The Most Common Method For Detecting Fraud? Â
The most common method is using rule-based systems, which employ predefined conditions to identify and respond to potential fraudulent activities.
Q4. What Algorithm Is Typically Used For Fraud Detection? Â
Machine Learning (ML) algorithms are commonly employed for fraud detection, providing adaptive learning capabilities to identify patterns and anomalies.
Q5. How Does Rule-Based Differ From ML-Based Fraud Detection? Â
Rule-based detection relies on predefined if/then logic, while ML-based detection uses adaptive algorithms to learn and identify patterns without explicit programming.
Streamline Compliance: Achieve 80% Faster Setup for Fraud Prevention

How Aseel reduced onboarding time by more than 87% using FOCAL
Learn how FOCAL empowered Aseel to achieve new milestones.
Mastering Fraud Prevention: A Comprehensive Guide for KSA and MENA Businesses
51% of organizations fell victim to fraud in the last two years, don't be caught off guard, act proactively.
Comments
Leave a Reply
Comment policy: We love comments and appreciate the time that readers spend to share ideas and give feedback. However, all comments are manually moderated and those deemed to be spam or solely promotional will be deleted.